Emotion recognition is the process of detecting a human’s emotion through several parameters such as body gestures, voice, text, and biological signs. As this world is moving to modernization, it is very difficult to recognize every individual’s emotions manually. “We are presenting you the most expected article in the area of emotion recognition using deep convolutional neural networks with thought-provoking concepts”
When using deep convolutional neural networks in emotion recognition, would offer unimaginable outcomes beyond your expectation. This article is all about that. This is article is popped up with a brief overview of emotion recognition with crisp points. Come let us sail with the handout’s flow!!!
Brief Overview of Emotion Recognition
Humans are expressing their emotions in diverse ways like text, voice (audio), gestures, and biological signs & mainly through facial expressions. Biological signs are subject to variations as the emotion changes along with it are consists of substantial emotions.
Though emotions are sensitive outcomes of human feeling thus it is very difficult to categorize them under a label as in the form of factors. Emotions can be expressed and recognized in 2 ways. One is human simply expresses their state of mind hence it is very easy for emotion recognition. Such as smile, joy, sad & angry.
Do you ever notice a person asking about your condition according to your facial feature condition or expression? Yes, almost every person faces these kinds of questions!!! These come under discrete labels. However, these labels cannot showcase the expression fusions.
In contrast to the discrete labels, biological signals unplanned expressions of the humans which could not be concealed. For instance, people cannot hide their anger when they are extremely burned out. However, people are tending to manipulate their conditions by changing their facial expressions.
In this regard, let us also discuss the real-time applications of emotion recognition. There are lots and lots of peoples’ states of mind is coded in different facial gestures and actions. We can assume a person’s condition by observing their facial expressions as stated in the upcoming section by the way of real-time applications.
Real-time Applications of Emotion Recognition
- Behavioral conditions such as unsociability, friendliness, nervousness
- Emotional statements about sicknesses for depression diagnosis
- Micro-expressions based concealed (hidden) emotions analysis
- Cognitive (reasoning) to detect either attention or inattentions
- State of emotional conditions such as anger, fear, happiness, etc.
The above listed are the various real-time applications of emotion recognition utilized. Apart from this, there are so many areas yet to cover and they are as you know very well. Besides, artificial intelligence is playing a huge role in detecting emotion and that is known as emotion artificial intelligence.
We know that deep learning & machine learning are the subsets of AI. In this manner, let us discuss how the inputs get processed under 2 of these techniques for your better understanding.
- Deep Learning
- Step 1: Input (image or text)
- Step 2: Simplest feature extraction
- Step 3: Multi-layers of abstract features
- Step 4: Mapping
- Step 5: Output
- Machine Learning
- Step 1: Inputs (image or text)
- Step 2: Manually crafted features
- Step 3: Automated feature extraction
- Step 4: Mapping
- Step 5: Output
The above listed are major techniques in which emotion is detected or recognized according to the inputs given. As this handout is titled with the emotion recognition using deep convolutional neural network, here we would like to state the 3 steps for detecting the emotion. The front image of the individual is used as the input in wide cases. Let’s have further discussions in the forthcoming section with brief explanations.
What are the Steps in Emotion Recognition?
- Image Processing
Facial features and regions are extracted in the image processing stage with the help of histogram, virtual or digital face model & fuzzy algorithms.
- Feature Extraction
Here, various facial features such as temporal and spatial features are extracted for the emotion detection process.
- Emotion Detection
Classifier techniques are proposed to detect or recognize human facial expression-based emotions in the extracted features.
Itemized above are the 3 major indispensable steps involved in the process of emotion recognition or detection. Our researchers in the concern are accustomed to the emotion detection processes. This is becoming possible by conducting habitual researches in the same areas. Here, you may get questions on what is the need behind detecting the emotions of a person. The next section is having answers. Let’s trespass this section to bring up the next.
Why is Emotion Detection Important?
The main objective behind emotion detection is to improve social relationships among people. Emotions can be replicated in various forms such as voice (tones), video, image, body gestures & even facial expressions.
When computerizing emotion detection, it needs huge interactions between the intellectual machines & human beings. For your better understanding, we are going to list out the various emotions of an individual with their feature conditions.
Emotions & Feature Conditions
- Joy
- Zygomatic
- Orbicularis
- Nose Labial Fold Formed
- Raised Upper Lips
- Sad
- Raised Eyebrow’s Inner Corners
- Depressed Mouth Corners
- Surprise
- Lips Swollen
- Raised Eyebrows
- Opened Mouth
- Widen Eyes
- Happy
- Under Eye Skin Wrinkles
- Wider Mouth Corners (Big Smile)
- Contempt
- Wrinkled Nose
- Lip Projections
- Raised Upper Lips
- Gazing Away
- Disgust
- Raised Upper Lips
- Downed Lower Lips
- Opened Mouth
- Anger
- Raised Nostrils
- Wider Eyes
- Reduced Mouth Corners
- Fear
- Raised Eyebrows
- Lips Compression
- Opened Mouth
- Widen Eyes
Above listed are the various emotions and the facial feature conditions according to the emotion expressed by an individual. As you know very well that, corporate and IT companies are investing a vast amount in review management.
However, the companies are not getting the appropriate state of mind (users). So that emotion recognition techniques are introduced and helping them a lot to detect the person behaviors under various circumstances with their emotions.
The major idea behind this technique is to detect and measure the reviews from customers in an eCommerce platform broadly. Many of the digital buyers are being asked to give their opinions and reviews of the product bought for improving their doings better in any other way. Now we can also learn how does artificial intelligence detects emotions with brief explanations.
How Does Artificial Intelligence Detect Emotions?
Artificial intelligence is widely used in emotion recognition or detection techniques and it is otherwise known as ‘affective computing. Humans use both nonverbal & verbal cues in communication to express their emotions.
An intellectual machine with emotional intelligence is highly capable of reasoning (cognitive) the inputs are given to it. Along with this, they are interpreting that image using recognizing the emotions of a person by their facial expressions and giving effective responses.
Intelligent machines are being inputted with a larger amount of inputs for detecting human emotions. Deep learning & machine learning are the sub-branches of artificial intelligence technology. In these (ML & DL) technologies, inputs are given as speech (voice) & images for interpreting with the same.
They effectively recognize the tone changes and expression changes. For example, if a person is screaming loud it may be considered as he is neither angry nor happy and even assumes the current state of the mind.
On the other hand, researchers are aimed to develop wearable technologies which have much intimacy with the users to detect the heart beat rates & some other parameters to detect the emotion of a person. There are so many biological signals are being used for emotion recognition in the form of solutions. Come, let us learn them.
Latest Solution for Emotion Recognition
- RSP – Respiration
- EOG – Electrooculography Signals
- SC – Skin Conductivity
- BVP – Blood Volume Pulse
- EMG – Electromyogram
- PPG – Photoplethysmogram
- EEG – Electroencephalogram
The aforementioned are some of the latest solutions (signals) for emotion recognition. Emotion recognition using deep convolutional neural networks is getting researched by so many researchers in recent eras.
Would you imagine a technology without intellectual skills? Emotion recognition is the system that is making it possible by using deep convolutional artificial neural network in their recognition processes. In this regard, next, we can have the section concreted with the steps in using DCNN.
Steps for Emotion Recognition using Deep Convolutional Neural Networks
- Step 1: Image Selection
- Step 2: Face Detection using Firebase API
- Face / Image Edges
- Step 3: Feature Extraction
- Grayscale Face Transformation
- Face Resizing (96*96 Matrix)
- Face Prediction using CNN
- Step 4: Emotion Classification
- Datasets
- Trained MobileNet V2
- Step 5: Emotion Prediction
The foregoing passage has conveyed to you the major 5 steps involved in the emotion recognition when it comes to deep convolutional neural networks. We are 10 dozen plus developers and researchers who are dynamically performing under various areas of technology.
So the skillsets of our researchers are enlarged day by day. Hence, our technical crew wanted to highlight the two different methods (feature extraction & selection) in emotion recognition for the ease of your understanding.
Feature Extraction and Selection Methods for Emotion Recognition
Different Features for Extraction
- Shape-based Features
- Index Shapes
- Irregularity
- Circularity
- Perimeter
- Area
- Texture oriented Features
- Local Binary Pattern
- Homogeneity
- Entropy & Energy
- Dissimilarity
- Cluster Shades
- Cluster Prominence
- Contrast & Correlation
- Auto Correlations
- Intensity oriented Features
- Pixel Angles
- Kurtosis
- Skewness
- Variance
- Average / Mean
The above listed are the various features used for the feature extraction processes according to several methods. For example, these features are extracted by deep learning-based convolutional neural networks.
As they extract features ranging from simple to high-level complex features automatically, it is widely preferred by many of the top engineers in the world. Come let us look into the feature extraction & selection methods for the ease of your understanding.
1. Feature Extraction Methods
- Feature Learning Methods
- Deep Learning Methods
- Bag of Features
- Textons
- Spatial Domain Methods
- Salient Color
- Local Color
- Color Histogram
- Color Moments
- Statistical Textures
- Local Binary Patterns
- Edge & Shape Features
- Geometric Texture & Color Features
- Frequency Domains
- Gabor Filter & Texture Analysis
- LBP based Wavelet Domains
- Statistical Texture Features
- Fourier Filter based Color Features
- DWT based Color Features
- Geometric Color & Texture Features
- Statistical Texture based Wavelet Domains
- GLCM based Color Texture
- Gabor based Color Texture
2. Feature Selection Methods
- Wrappers
- Gabor Filters
- Feature Subset Search Algorithms
- Search Organization
- Feature Generation
- Feature Evaluation
The above-itemized methods are accustomed to some of the techniques to boost up their process in an optimum manner. There are so many methods used for emotion recognition. Like the feature extraction and selection methods, deep learning & machine learning methods are also used by many of the developers. Such methods are covered in the next section.
Deep Learning Methods for Face Emotion Recognition
- Supervised Methods
- Recurrent Neural Network
- Deep Neural Network
- Convolutional Neural Network
- Unsupervised Methods
- Generative Adversarial Network
- Deep Belief Network
- Auto Encoder
The aforementioned are the irreplaceable methods used for emotion recognition and hybrid methods are the combination of both methods. As this article is focused on giving emotion recognition using deep convolutional neural networks, we wanted to list out the different types of DCNN for your better understanding.
Different Types of DCNN for Emotion Recognition
- Width based Multi-connection CNN
- Xception
- ResNet
- Inception Family
- Pyramidal Net
- WideResNet
- Attention-based CNN
- Concurrent Squeeze & Excitation
- Residual Attention Neural Network
- Convolutional Block Attention
- Channel Exploitation based CNN
- Channel Boosted CNN (TL)
- Feature-map Exploitation based CNN
- Competitive Squeeze & Excitation
- Squeeze & Excitation
- Multi-path based CNN
- DenseNet
- Highway Nets
- ResNet
- Depth based CNN
- Inception-ResNet
- Inception V3 & V4
- Spatial Exploitation based CNN
- ZfNet
- GoogleNet
- VGG
- AlexNet
- LeNet
These are the various types of deep convolutional neural networks for emotion recognition. On the other hand, it is very important to evaluate the performance of the system by several metrics and such metrics are comprised in the following passage. Look into the next section to grab the performance metrics of emotion recognition.
What are the Performance Metrics for Emotion Recognition?
- Emotional Response
- Recognition Accuracy
Listed above are the two major metrics, mainly used for evaluating the performance of emotion recognition. There are so many datasets are being used to recognize the emotion of human beings. Among those datasets, here we are going to illuminate some of the essential datasets for the ease of your understanding.
Datasets for Face Emotion Recognition
- BU-3DFE
- Description: Extracts 3D Images & Consists of 6 Expressions
- No.of Sequences: 2500
- Inputs: Texts & Images
- Used for: Facial Expression Recognition & Classification
- Cohn-Kanade AU-Coded Expression Database
- Description: Consists of Expression based Labeled Images
- Tasks: Facial Feature Tracking
- No.of Sequences:
- Inputs: Texts & Images
- Used for: Facial Expression Analysis
- JAFFE Facial Expression Database
- Description: Consists of Japanese Models’ 213 Images with 7 Expressions
- Tasks: Image Cropping & Semantic Rating
- No.of Sequences: 213
- Inputs: Texts & Images
- Used for: Facial Expression Cognition
These are the essential datasets being used in facial emotion recognition. So far, we have come up with all the needed areas of emotion recognition using deep convolutional neural networks. In addition, we hope that you would have understood the concepts as of now listed.
“Finally, we are wishing you people to yield best results in these areas by conducting researches”
Subscribe Our Youtube Channel
You can Watch all Subjects Matlab & Simulink latest Innovative Project Results
Our services
We want to support Uncompromise Matlab service for all your Requirements Our Reseachers and Technical team keep update the technology for all subjects ,We assure We Meet out Your Needs.
Our Services
- Matlab Research Paper Help
- Matlab assignment help
- Matlab Project Help
- Matlab Homework Help
- Simulink assignment help
- Simulink Project Help
- Simulink Homework Help
- Matlab Research Paper Help
- NS3 Research Paper Help
- Omnet++ Research Paper Help
Our Benefits
- Customised Matlab Assignments
- Global Assignment Knowledge
- Best Assignment Writers
- Certified Matlab Trainers
- Experienced Matlab Developers
- Over 400k+ Satisfied Students
- Ontime support
- Best Price Guarantee
- Plagiarism Free Work
- Correct Citations
Expert Matlab services just 1-click
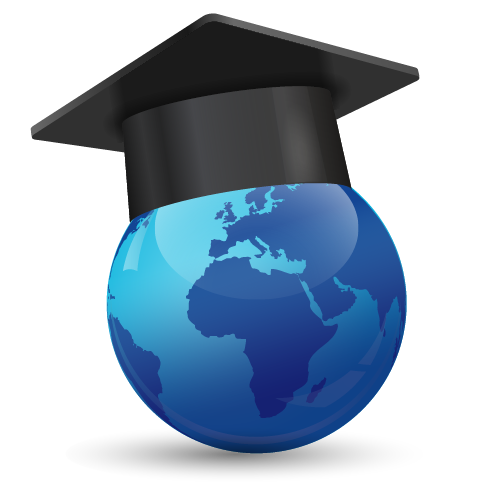
Delivery Materials
Unlimited support we offer you
For better understanding purpose we provide following Materials for all Kind of Research & Assignment & Homework service.
Programs
Designs
Simulations
Results
Graphs
Result snapshot
Video Tutorial
Instructions Profile
Sofware Install Guide
Execution Guidance
Explanations
Implement Plan
Matlab Projects
Matlab projects innovators has laid our steps in all dimension related to math works.Our concern support matlab projects for more than 10 years.Many Research scholars are benefited by our matlab projects service.We are trusted institution who supplies matlab projects for many universities and colleges.
Reasons to choose Matlab Projects .org???
Our Service are widely utilized by Research centers.More than 5000+ Projects & Thesis has been provided by us to Students & Research Scholars. All current mathworks software versions are being updated by us.
Our concern has provided the required solution for all the above mention technical problems required by clients with best Customer Support.
- Novel Idea
- Ontime Delivery
- Best Prices
- Unique Work
Simulation Projects Workflow

Embedded Projects Workflow
