GENETIC ALGORITHM PROJECTS
The term genetic algorithm is used as search technique to calculate the true or relevant solution and search problems. A computational problem to carry out task effectively in the changing atmosphere.A computing technique for processing evolutionary method is genetic algorithm. GENETIC ALGORITHM PROJECTS provides answer for chromosomes by bit coding and search for good solution candidate in space genotype by using selection, mutation and crossover which are the GA operations.
To produce higher recognition and accurate classification genetic algorithm projects are developed in matlab simulation. Intention of population is an important concept in GA. Population size is a user-specified parameter and is an important factor that affects the performance of genetic algorithms and scalability.Problems in genetic algorithms are nonlinear. Each parameter must be treated as independent variable and can be solved independent from other variables.
2015 IEEE Genetic Algorithm Projects
- Optimizing Template for Lookup-Table Inverse Halftoning using Elitist Genetic Algorithm.
- Genetic Algorithm-Based Classifiers Fusion for Multisensor Activity Recognition of Elderly People.
- Optimal scheduling for maintenance period of generating units using a hybrid scatter-genetic algorithm.
- Optimal Electromagnetic Design of a Nonsalient Magnetic-Cored Superconducting Synchronous Machine Using Genetic Algorithm.
- A Genetic-Algorithm-Based Approach to Solve Carpool Service Problems in Cloud Computing.
- ASMiGA: An Archive-Based Steady-State Micro Genetic Algorithm.
- Feature Selection Based on Hybridization of Genetic Algorithm and Particle Swarm Optimization.
- Magnetic Material Group Furnace Problem Modeling and the Specialization of the Genetic Algorithm.
- Polarization-Engineered High-Efficiency GaInN Light-Emitting Diodes Optimized by Genetic Algorithm.
- Real coded genetic algorithm approach with random transfer vectors-based mutation for short-term hydro–thermal scheduling.
- Empirical Forecasting of HF-Radar Velocity Using Genetic Algorithms.
- Characteristics Optimization of the Maglev Train Hybrid Suspension System Using Genetic Algorithm.
- Electric Vehicle Route Optimization Considering Time-of-Use Electricity Price by Learnable Partheno-Genetic Algorithm.
- Discussion on “A Genetic Algorithm-Based Low Voltage Ride-Through Control Strategy for Grid Connected Doubly Fed Induction Wind Generators”.
- Closure to Discussion on “A Genetic Algorithm-Based Low Voltage Ride-Through Control Strategy for Grid Connected Doubly Fed Induction Wind Generators”.
Motivation of genetic algorithm is an effective theory where biological riles like selective breeding and common descent is used for human benefit.
Steps Genetic Algorithm Projects: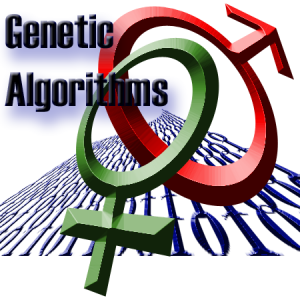
Mutation:
The types of operator used in neighborhood search and its extensions that are nearing to the concept is mutation operators by adding Gaussian noise mutation of an real number is recognized, the parameters of Gaussian is controlled by ES allowing distribution coverage to global optimum.
Selection:
To emphasize good solution and cancel the bad solution in population by not altering the population size is the prime objective of selection operators.
Crossover:
Selecting genes from parent chromosomes by creating new offspring is crossover. The two parents are recombined to form new offspring where the chromosomes of two parents are transferred to next generation.
PhD scholars could carry out the genetic algorithm projects as per their requirements. Paper title for genetic algorithm projects are updated from Scopus journals which has high impact factor.
Approaches to encode Genetic algorithm Projects:
Tree Encoding:
The chromosome is represented in tree structure.
Binary Encoding:
Each chromosome is a string of 0 or 1.
Value Encoding:
A chromosome is a sequence of values.
Permutation Encoding:
A chromosome is a string of numbers which represent position in a sequence.
Methods used in Genetic Algorithm projects
- Methods of Reproduction.
- Methods of Representation.
- Methods of Selection.
Selection methods are,
- Elitist Selection.
- Roulette-wheel selection.
- Scaling selection.
- Rank selection.
- Fitness-proportionate selection.
Subscribe Our Youtube Channel
You can Watch all Subjects Matlab & Simulink latest Innovative Project Results
Our services
We want to support Uncompromise Matlab service for all your Requirements Our Reseachers and Technical team keep update the technology for all subjects ,We assure We Meet out Your Needs.
Our Services
- Matlab Research Paper Help
- Matlab assignment help
- Matlab Project Help
- Matlab Homework Help
- Simulink assignment help
- Simulink Project Help
- Simulink Homework Help
- Matlab Research Paper Help
- NS3 Research Paper Help
- Omnet++ Research Paper Help
Our Benefits
- Customised Matlab Assignments
- Global Assignment Knowledge
- Best Assignment Writers
- Certified Matlab Trainers
- Experienced Matlab Developers
- Over 400k+ Satisfied Students
- Ontime support
- Best Price Guarantee
- Plagiarism Free Work
- Correct Citations
Expert Matlab services just 1-click
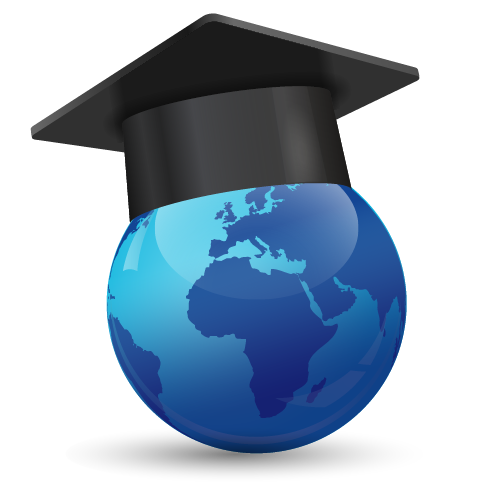
Delivery Materials
Unlimited support we offer you
For better understanding purpose we provide following Materials for all Kind of Research & Assignment & Homework service.
Programs
Designs
Simulations
Results
Graphs
Result snapshot
Video Tutorial
Instructions Profile
Sofware Install Guide
Execution Guidance
Explanations
Implement Plan
Matlab Projects
Matlab projects innovators has laid our steps in all dimension related to math works.Our concern support matlab projects for more than 10 years.Many Research scholars are benefited by our matlab projects service.We are trusted institution who supplies matlab projects for many universities and colleges.
Reasons to choose Matlab Projects .org???
Our Service are widely utilized by Research centers.More than 5000+ Projects & Thesis has been provided by us to Students & Research Scholars. All current mathworks software versions are being updated by us.
Our concern has provided the required solution for all the above mention technical problems required by clients with best Customer Support.
- Novel Idea
- Ontime Delivery
- Best Prices
- Unique Work
Simulation Projects Workflow

Embedded Projects Workflow
